The Data Science Behind Brandwatch’s New Sentiment Analysis
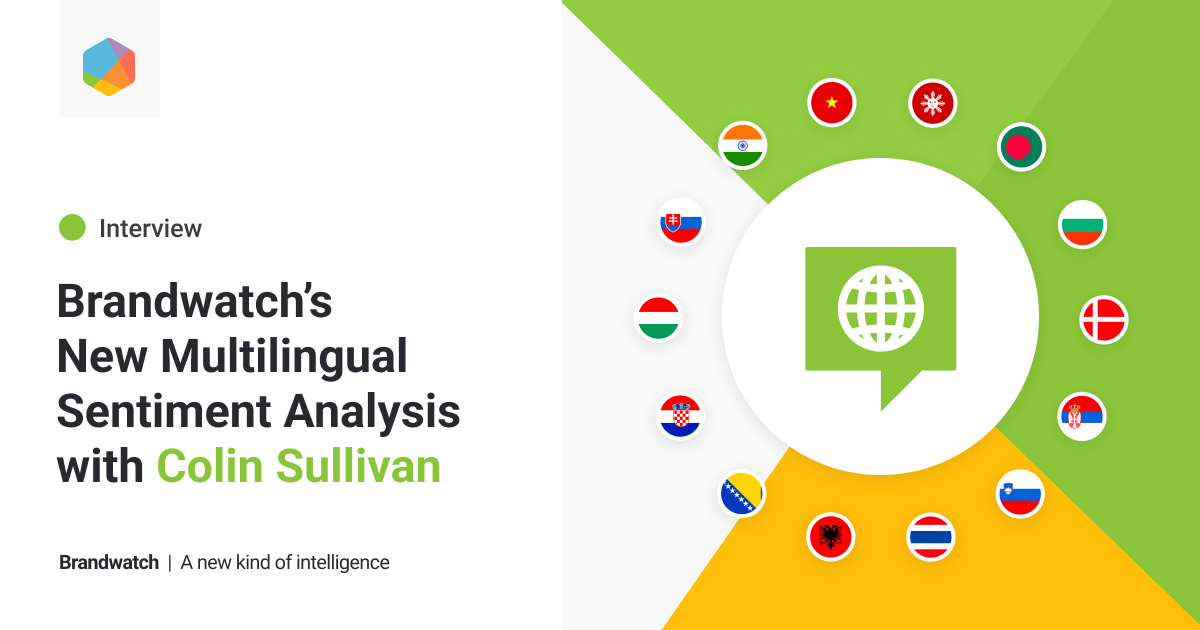
[ad_1]
This month Brandwatch rolled out a whole new sentiment model across the more than 100m online sources we cover in Brandwatch Consumer Research as well as the apps powered by Brandwatch like Cision Social Listening and Falcon Listen.
It is a big upgrade on Brandwatch’s existing world-class sentiment analysis, providing around 18% better accuracy on average across previously supported languages.
This new model is also multilingual, meaning:
- Official support has been added for 16 new evaluated languages, with more to come (bringing the current total of officially supported languages to 44)
- The model will also attempt to assign sentiment to posts in any other language (and posts with no language identified, like emoji-only posts) when it is confident enough
Sentiment is one of the key metrics Brandwatch customers rely on for a number of important tasks such as:
- Assessing brand health
- Identifying advocates or detractors
- Detecting emerging crises
- Understanding positive and negative topics related to brand or topic conversations
I sat down with one of the data scientists leading the team who developed our new sentiment model, Colin Sullivan, to ask him how it works and how it will benefit Brandwatch customers.
Hi Colin! We’re really excited to be able to see the fruits of your labour now available in Brandwatch’s sentiment analysis. Before we talk about this new sentiment model, tell us a little about yourself and your background.
Thanks Nick, we’re excited too! I’m a Data Science Manager leading several different projects here at Brandwatch and my background is in linguistics and computational linguistics.
Linguistics is essentially a social science involved in figuring out the patterns and rules that govern how language works looking at the theoretical background, syntax, and semantics of language.
Computational linguistics is the study of how computers can model these same structures and apply these models to things like Natural Language Processing, language identification, and how things get indexed. And it is also used to analyze things like sentiment and topics within large volumes of text data.
This sentiment update uses an entirely new model. Why build a new way of analyzing sentiment?
Two key reasons.
1. We wanted to make a jump to some of the state-of-the-art methods emerging in the research world. There have been some really exciting new developments in recent years that can help us achieve even better results.
2. We also saw an opportunity to simplify how we do sentiment at Brandwatch. We used to do the same procedure for each language that we supported which involved gathering a whole bunch of training data for each language, getting it labelled, learning about its linguistic patterns and then building a supervised learning model for each one. Moving to this new setup we have a single methodology that works for many languages at once.
This new model uses ‘transfer learning’. What is that exactly?
Over the last few years, the field of AI has made exciting progress with transfer learning which basically involves first training a model to have a more general understanding and then transferring that learning and asking it to apply it to a different task. This is very different to training a model only to solve a single, specific problem, which is how we used to do sentiment analysis.
So our new model has first been trained to have a general sense of how language is used. We then take a secondary step to point that model at a task like sentiment analysis.
The first step is very similar to how next-word auto suggestion works. A model with enough experience of language being used by humans can start to predict what the next words are likely to be if you give it some text. Next, we ask it to ‘predict’ a topic that encapsulates the meaning of a whole sentence or social media post, in this case the topics are ‘positive’, ‘negative’ or ‘neutral’ – it re-uses all the same information from step one.
This is actually how your brain works when you listen to someone talk. You are, subconsciously, constantly trying to predict what they are going to say next in order to better hear and understand them.
How does this help Brandwatch define sentiment better than before?
One of the key advantages of this new approach is that it makes it more robust when dealing with more complex or nuanced language. The new model can see past things like misspellings or slang.
Previously, supervised learning models would be restricted to a fixed set of known patterns during training, which did not come close to exhaustively capturing all linguistically plausible ways of expressing a concept. New state-of-the-art models are better able to re-use what it already knows when faced with new or rare patterns.
The transfer learning approach means the model will take what it knows to fill in gaps. For example, it can break down words it doesn’t know into parts that might give it clues (just like you would!).
And it works in almost any language because we are not training for a new language each time. This also means it can handle a wider range of regional dialects and posts where someone switches between languages.
[ad_2]
Source link